Knowledge Graph is a powerful asset… but it still needs to be mastered. What challenges arise from this technology ?
In our previous article, we presented Knowledge Graphs. A technology capable of mapping a global knowledge network between people.
This article introduces the basic notions behind Knowledge Graphs. We warmly invite you to read it before continuing.
In this second part, we would like to share with you the technical challenges that must be overcome to fully master Knowledge Graphs.
Knowledge Fusion
First challenge is to answer a simple question.
How to create a World Knowledge Graph without redundancy, without having duplicates ?
Let me explain. When you search through the web for a person, let’s say John Smith, this person surely has several social networks: Instagram, Linkedin, Facebook, etc.
How do you determine that these accounts belong to the same person ?
Especially since there are surely several John Smiths on Earth.
So how to build an algorithm understanding that the two John Smith accounts on Facebook are different people but that these two other accounts on Instagram belong to the same one ?
More globally, how do you capture all the information on the Web to build an unbiased database ?
This challenge is called Knowledge Fusion.
The idea is to gather all the knowledge on the Internet while avoiding redundancy as well as losing information !
Since we are only at the beginning of this technology very few solutions exist.
Only one is mentioned during Mike Tung’s interview, the Record Linkage Model💥
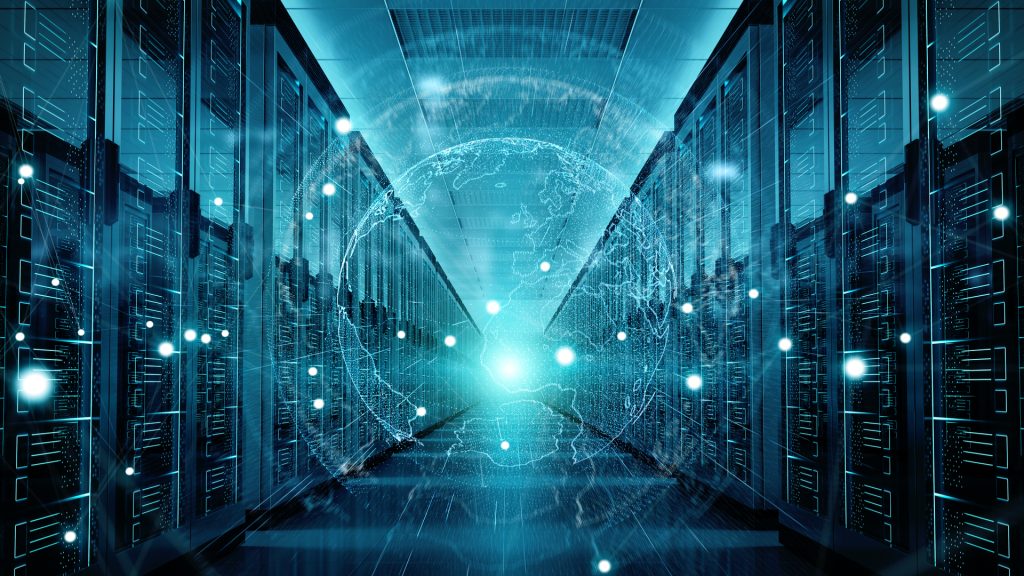
Storage and Computing Power
Here we approach a more familiar problem… but massively amplified by the very nature of Knowledge Graphs.
Data storage problem.
Indeed this problem exists in most businesses… so imagine for a company that wants to store the entire Web !
On top of that, you have to add the computing power needed to perform these operations.
This issue is omnipresent in our world today.
Moreover, not only in the business world.
For example, graphics cards and SSDSs are being snapped up at a premium today, because the materials needed to produce them are not unlimited.
So between crypto-currency miners, Cyberpunk 2077 gamers and entrepreneurs who want to crawl the web, which ones will win the battle for Computing Power ? 🥇
Graph Embedding
Actually, the previous problem is not a question of technological advancement but more a problem of cost.
It does not require a technical innovation but only an economic investment.
No, the real challenge is that once you have stored the Web, you need to be able to explore it efficiently.
Indeed, the main goal of a Knowledge Graph is to deliver clear and precise information to its user.
By the way, if your goal is to master Deep Learning - I've prepared the Action plan to Master Neural networks. for you.
7 days of free advice from an Artificial Intelligence engineer to learn how to master neural networks from scratch:
- Plan your training
- Structure your projects
- Develop your Artificial Intelligence algorithms
I have based this program on scientific facts, on approaches proven by researchers, but also on my own techniques, which I have devised as I have gained experience in the field of Deep Learning.
To access it, click here :
Now we can get back to what I was talking about earlier.
But even if this answer will perfectly match the user’s need, if it takes 10 years to be found, it loses its usefulness.
So, we need to find a quick way to explore the Knowledge Graph.
In other words, we need to find a way to efficiently structure the information stored in the Graph.
This problem already exists in Deep Learning.
In particular, to analyze text, we use an Embedding layer. This allows to transcribe sentences into a list of numbers.
It is thus much faster for a computer to perform calculations on a list of numbers than on a list of words.
In a way, we encode the sentence so that the computer can better understand and analyze it (more information on Text Preprocessing is provided in this article 😉 ).
One of the challenges of Knowledge Graphs is therefore to find an efficient Embedding layer to transcribe all the information about an individual: his activities, his relationships, the things he likes, …
The list is endless, and that’s the challenge !
It is not as simple as encode a sentence of 5 or 20 words, here we need to embed all the information that a person or an entity has on the Web.
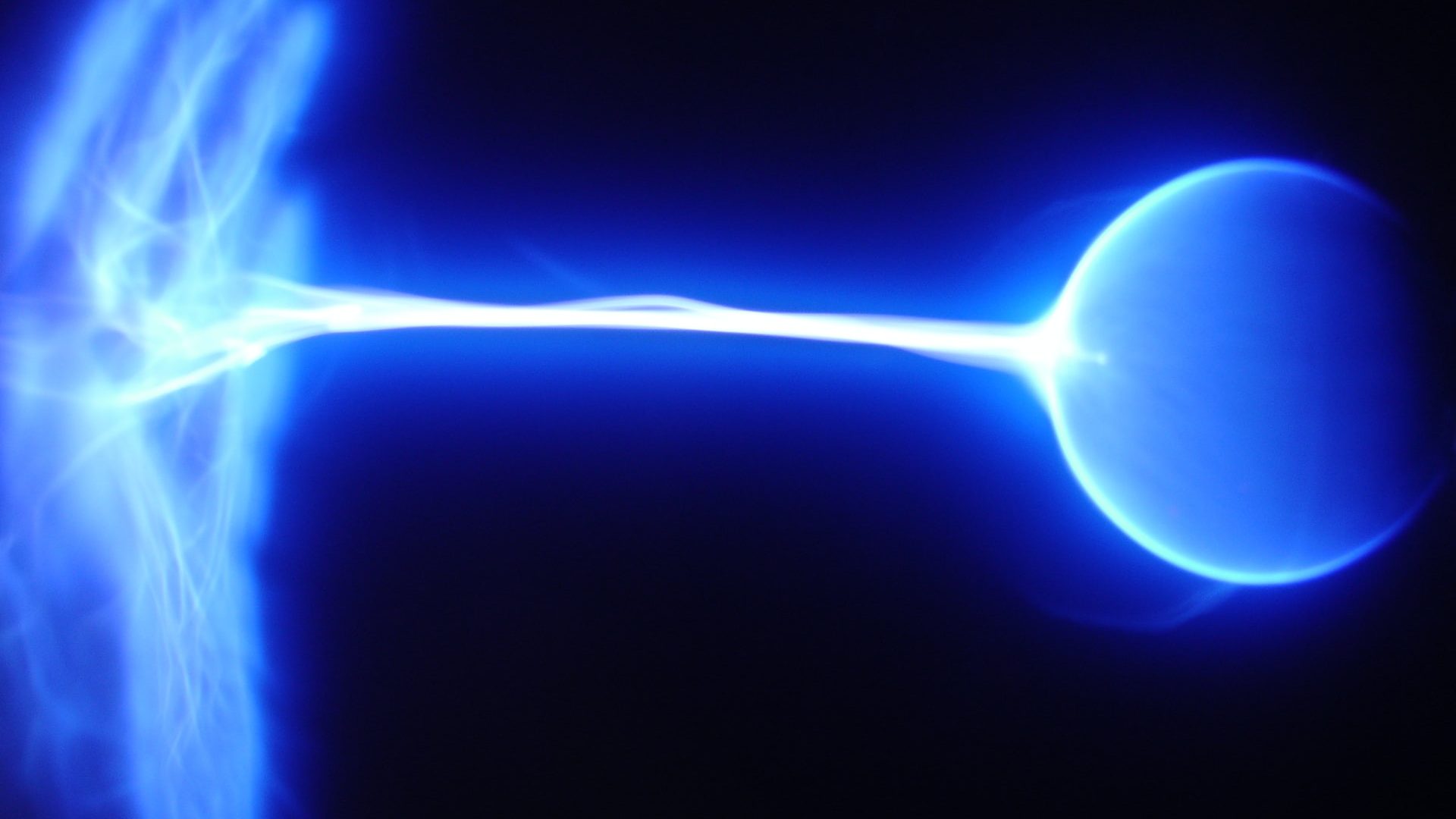
No Unified Theory
We have reached the last challenge of Knowledge Graphs and it is, according to me, the major one that will enable us to address the problems explained above.
This challenge is the absence of Theory on the subject.
In fact, since Knowledge Graphs are a fairly recent subject, or at least the possibility of creating them is new, there is very little research on the topic.
And furthermore, since the construction of a Knowledge Graph based on the Web is not within the reach of everyone, very few researchers can really study it.
On the one hand, there is a lack of theory that makes the creation of this technology more complex and on the other hand, a lack of access to information that prevents it from being studied.
In fact, this challenge is well known in the scientific world : finding investors to make research progress.
Diffbot, Mike Tung’s company, is announcing that it will soon share an open source reference dataset to build our own knowledge graphs from texts.
He also wants to invest in research and wants to collaborate with universities in this perspective.
But the question is always the same for the Private Sector: will it bring money in the future ? 💰
And more than that… what will Knowledge Graphs look like in 5 years ? Will they be beneficial to society ? How will they be used ? See you in the last part of this article to find out !
sources :
- Towardsdatascience – Mike Tung Interview
- Photo from Cyber-Prog
One last word, if you want to go further and learn about Deep Learning - I've prepared for you the Action plan to Master Neural networks. for you.
7 days of free advice from an Artificial Intelligence engineer to learn how to master neural networks from scratch:
- Plan your training
- Structure your projects
- Develop your Artificial Intelligence algorithms
I have based this program on scientific facts, on approaches proven by researchers, but also on my own techniques, which I have devised as I have gained experience in the field of Deep Learning.
To access it, click here :