In the scientific world of Deep Learning, GAN are increasingly attracting attention. But what are they in practice ?
Generative Antagonistic Networks (GAN) is a barbaric name for Deep Learning models that generate information, images, music.
It is a type of Neural Network which we have not yet discussed at Inside Machine Learning and yet, it has an increasingly important place in scientific research in Artificial Intelligence.
That’s why we invite you to explore Generative Antagonistic Networks now in this post !
The structure of GANs
GAN are category of neural network based on unsupervised learning algorithms.
This means that the network trains itself without a human previously giving it a goal.
To understand how they work, let’s simply decode their names: GAN
A GAN is a Generative Antagonist Network.
The terms “network” and “generative” are quite obvious.They are Neural Networks like CNN or RNN but which are used here to generate, to create things and not only to detect. It is therefore a generative network.
But then why do we talk about an Antagonist ?
In movies, an Antagonist is the opponent of the Protagonist: the main hero. We therefore say that the two characters are antagonists, meaning that they are opposites, rivals.
In other words, a story contains two characters who each have an opposite goal : defeat the other, achieve victory.
What does this have to do with Deep Learning ? Rest assured, it is part of the explanation 😎
By the way, if your goal is to master Deep Learning - I've prepared the Action plan to Master Neural networks. for you.
7 days of free advice from an Artificial Intelligence engineer to learn how to master neural networks from scratch:
- Plan your training
- Structure your projects
- Develop your Artificial Intelligence algorithms
I have based this program on scientific facts, on approaches proven by researchers, but also on my own techniques, which I have devised as I have gained experience in the field of Deep Learning.
To access it, click here :
Now we can get back to what I was talking about earlier.
In fact in a GAN, like in a movie, there are Antagonists. Only here, instead of having two characters competing for victory, we have two Neural Networks competing to optimize each other !
The Antagonistic Networks
But how do the two Antagonistic Networks of a GAN operate ?
In fact, they each have a very distinct role.
Let’s take the case of a GAN applied to images (the most frequent case).
One of the networks will train on a dataset and try to reproduce and generate images by faking the original ones.
Its antagonist, on the other hand, will analyze all the images (fake as well as real) and detect those that they consider as fake.
The more the antagonist detects fake images, the more the generative network will have to improve itself in faking images.
In fact, the goal of a GAN is to make the faker more efficient than the detector !
At the end of the training, the GAN must be able to generate images that are faithful to reality. To achieve this, it must fool the antagonist.
Here is a concrete example of a GAN result that transforms horses image into zebras:
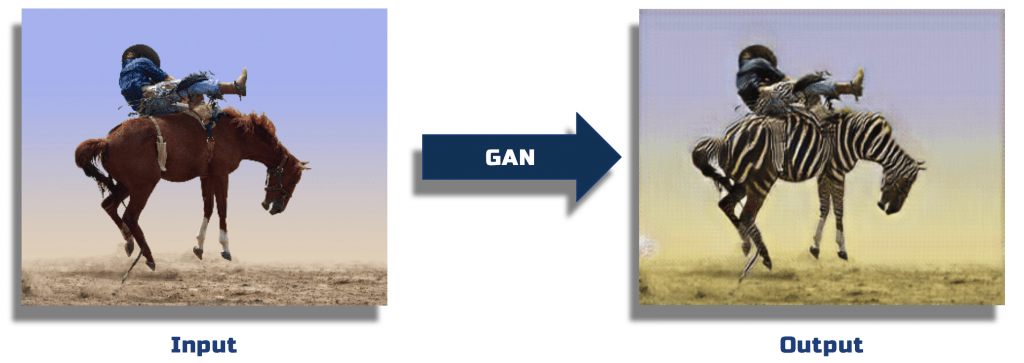
That’s the end of this article, we hope it helped you to better understand Generative Antagonistic Networks.
We’ll see you shortly in a tutorial to discuss in detail how to code a GAN!
sources :
- Photo by Ray Hennessy on Unsplash
One last word, if you want to go further and learn about Deep Learning - I've prepared for you the Action plan to Master Neural networks. for you.
7 days of free advice from an Artificial Intelligence engineer to learn how to master neural networks from scratch:
- Plan your training
- Structure your projects
- Develop your Artificial Intelligence algorithms
I have based this program on scientific facts, on approaches proven by researchers, but also on my own techniques, which I have devised as I have gained experience in the field of Deep Learning.
To access it, click here :
Generative adversarial
networks (GANs)