How does an Encoder-Decoder work and why use it in Deep Learning?
The Encoder-Decoder is a neural network discovered in 2014 and it is still used today in many projects.
It is a fundamental pillar of Deep Learning.
It is found in particular in translation software. This is the case, for example, of the neural network at the origin of Google Translation.
It is therefore used widely for NLP tasks (text processing), but it can also be used for Computer Vision!
This makes it an essential architecture to know.
What is an Encoder-Decoder ?
Encoder-Decoder is a neural network.
Or rather, it is a Deep Learning model composed of two neural networks.
These two neural networks usually have the same structure.
The first one will be used normally but the second one will work in reverse:
- A first neural network will take a sentence as input to output a sequence of numbers.
- The second network will take this sequence of numbers as input to output a sentence this time!
In fact these two networks do the same thing. But one is used in the normal direction and the other in the opposite.
So we have a sentence, a sequence of words, which is encoded into a sequence of numbers, then decoded into another sequence of words : the translated sentence.
The first neural network is called the encoder and the second neural network the decoder.
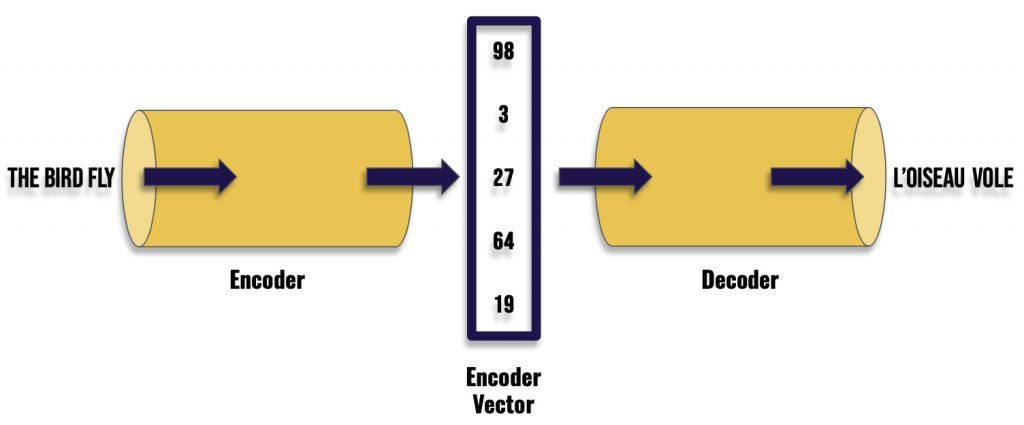
But why is the Encoder-Decoder effective for translation ?
It is difficult (and unreliable) to use classical neural network to go from a French sentence to its English translation.
By the way, if your goal is to master Deep Learning - I've prepared the Action plan to Master Neural networks. for you.
7 days of free advice from an Artificial Intelligence engineer to learn how to master neural networks from scratch:
- Plan your training
- Structure your projects
- Develop your Artificial Intelligence algorithms
I have based this program on scientific facts, on approaches proven by researchers, but also on my own techniques, which I have devised as I have gained experience in the field of Deep Learning.
To access it, click here :
Now we can get back to what I was talking about earlier.
Indeed, if we translate a sentence directly with the classical approach, the network would translate word by word without caring about the global meaning of the sentence.
For example, if we have the sentence “prendre une expression au pied de la lettre” and we translate it word by word, we would obtain: “take an expression at the foot of the letter”. It doesn’t mean anything in English.
But the Encoder Decoder approach solves this problem.
Indeed, the structure of the encoder allows to extract the meaning of a sentence.
It stores the extracted information in a vector (the result of the encoder).
Then, the decoder analyzes the vector to produce its own version of the sentence.
In our previous example, the Encoder-Decoder would be closer to the actual translation: “take an expression literally”.
This result is much better!
It is thanks to the Encoder that we can extract the meaning of a sentence. This information is then encoded in the form of a vector that the decoder can then use efficiently.
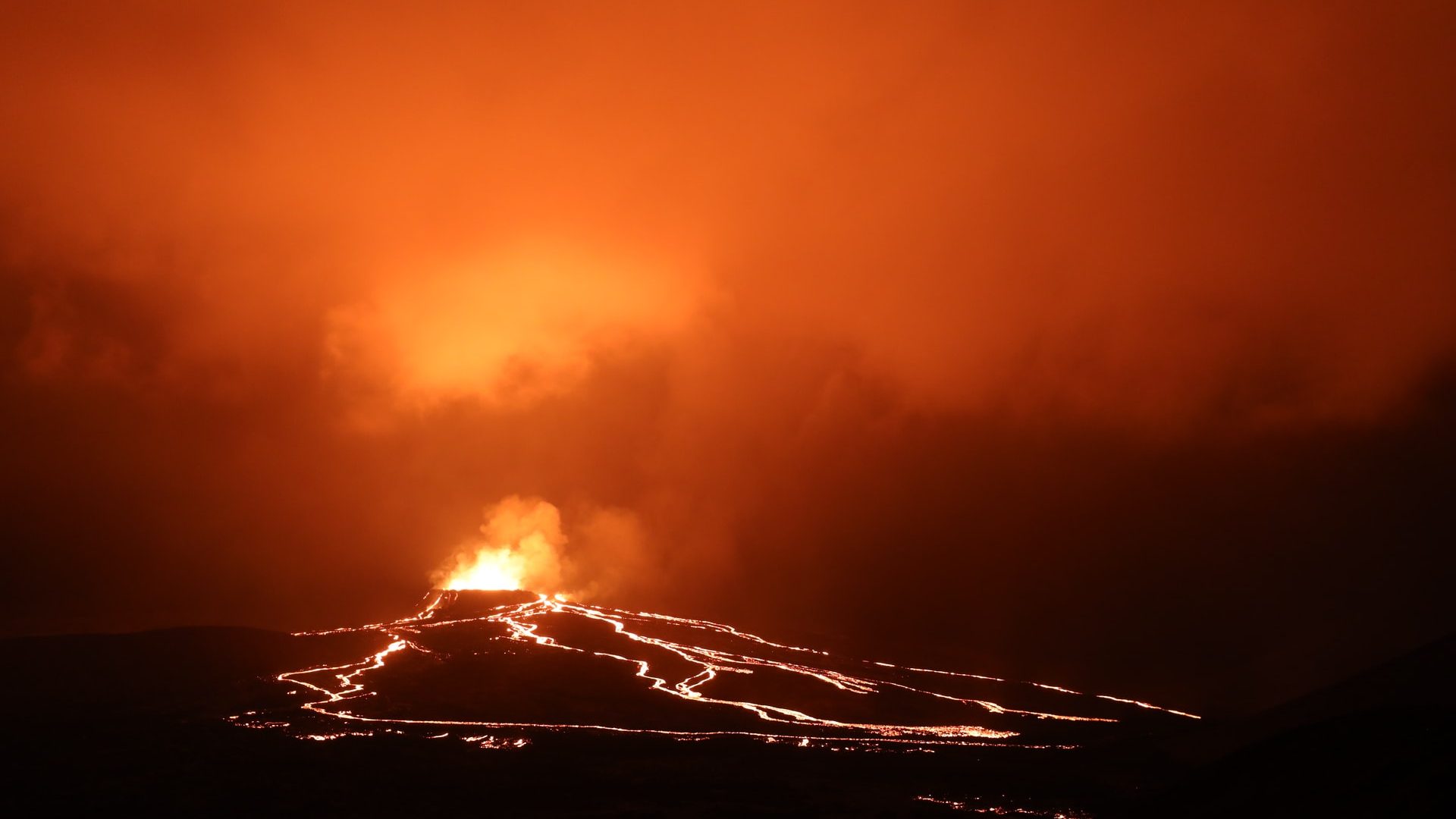
Conclusion
Encoder-Decoders are widely used in the research world, but they have a flaw.
The vector that the Encoder produces is fixed.
It will therefore be efficient for tasks where the sentence is small but as soon as the sentence is long, the vector will not be able to store the necessary information.
In this specific case, the final translation may not be relevant.
Fortunately for us, Encoder-Decoders are now coupled with mechanisms that allow them to adapt to sentences of any size
I talk about it in my article on the attention mechanism!
One last word, if you want to go further and learn about Deep Learning - I've prepared for you the Action plan to Master Neural networks. for you.
7 days of free advice from an Artificial Intelligence engineer to learn how to master neural networks from scratch:
- Plan your training
- Structure your projects
- Develop your Artificial Intelligence algorithms
I have based this program on scientific facts, on approaches proven by researchers, but also on my own techniques, which I have devised as I have gained experience in the field of Deep Learning.
To access it, click here :
Suppose that we use neural networks to implement the encoder–decoder architecture. Do the encoder and the decoder have to be the same type of neural network?